Efficiently targeting local consumer preference
Pinpointing localized consumer preferences has always been a challenge. While predictive and descriptive intelligence could match plans with local customer requirements, how can companies uncover hidden patterns and trends without drowning in the data or employing an army of analysts?
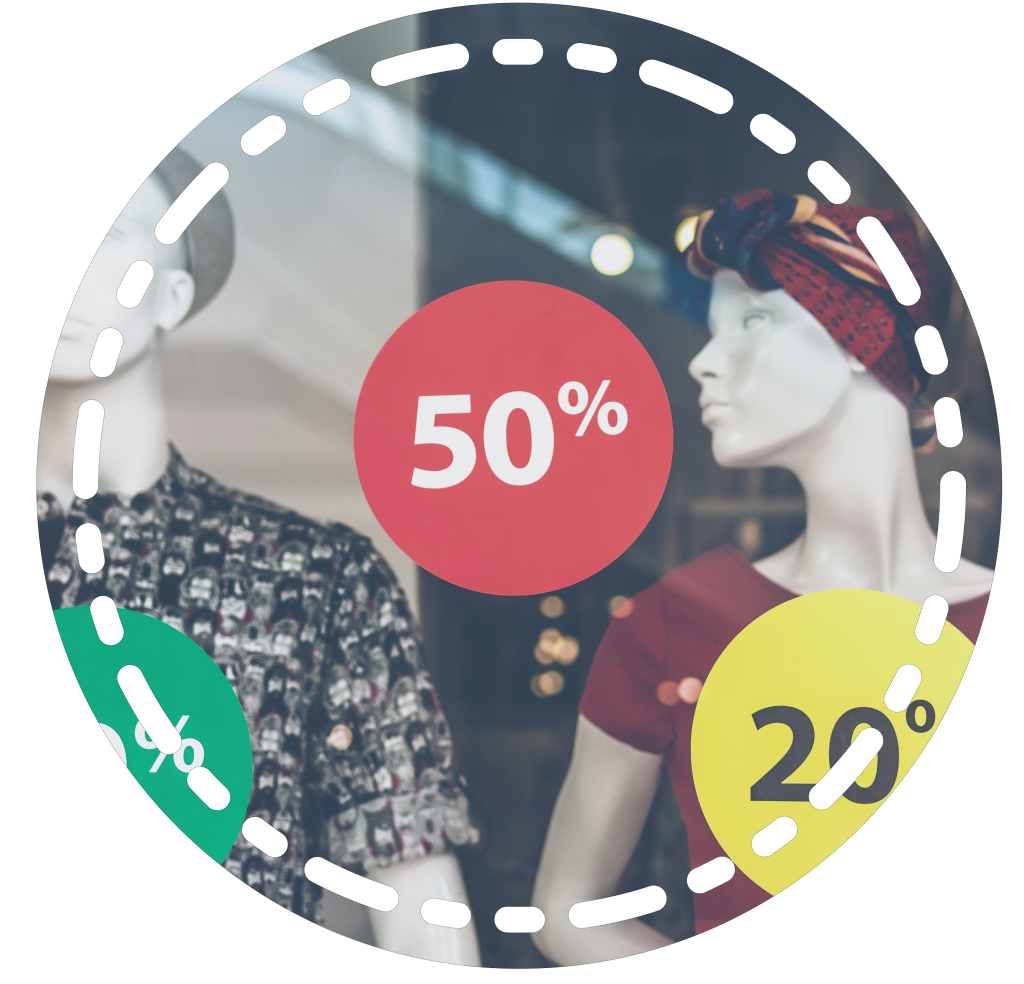
Cluster stores based on key performance indicators
provide a different store clustering for each major category within your store and correlates groups based on driving indicators store attributes, item attributes, store area etc
Deliver localized assortments with Channel Clustering
quickly determine which stores exhibit similar consumer preference patterns for a category, cluster them into store groups and then assort by cluster. By planning assortments for each group with similar product preferences, you’ll reduce unproductive inventory while fulfilling local demands.
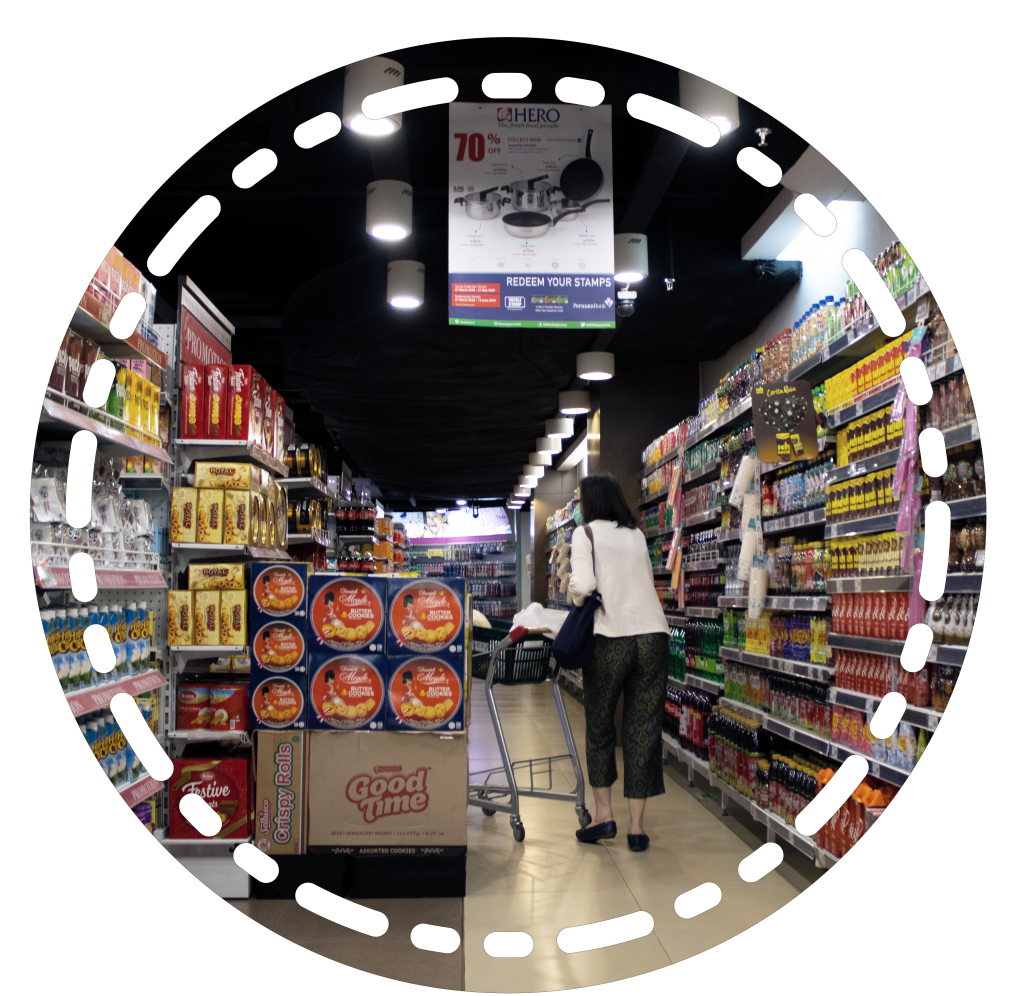
Make better category decisions at the shelf and floor
Enables your planners to target preferred local product demand in terms of popularity and shelf inventory. The application also helps to find the optimal number of groups to balance your micro-merchandising goals with your operational costs to save valuable time and money.
Optimize channel planning efforts
form unique store groups by product segment (i.e., class, department and category). Plus you can plan by representative group instead of individual stores. You’ll produce the optimum number of plans needed to achieve spot-on merchandising results within every channel.
Key Benefits

Establishes consumer preference patterns based on analysis of historical performance such as category POS transactions or opportunity forecast data

Draws from any performance measure to drive clustering (units, sales, turns, GMROI, etc.)

Pinpoints driving characteristics within a group, such as size and type of store, volume or type of merchandise sold, customer and item attributes and store trade area

Supports flexible management of store group outliers and new stores using automated or manual assignment procedures

Filters out extreme outliers and other anomalies when establishing preference patterns

Supports dynamic grouping of store locations based on performance or attribute information